Rev Launches Tools to Improve Automatic Speech Recognition Benchmarking
Rev is excited to announce the release of two free tools to help customers and researchers test automatic speech recognition accuracy.
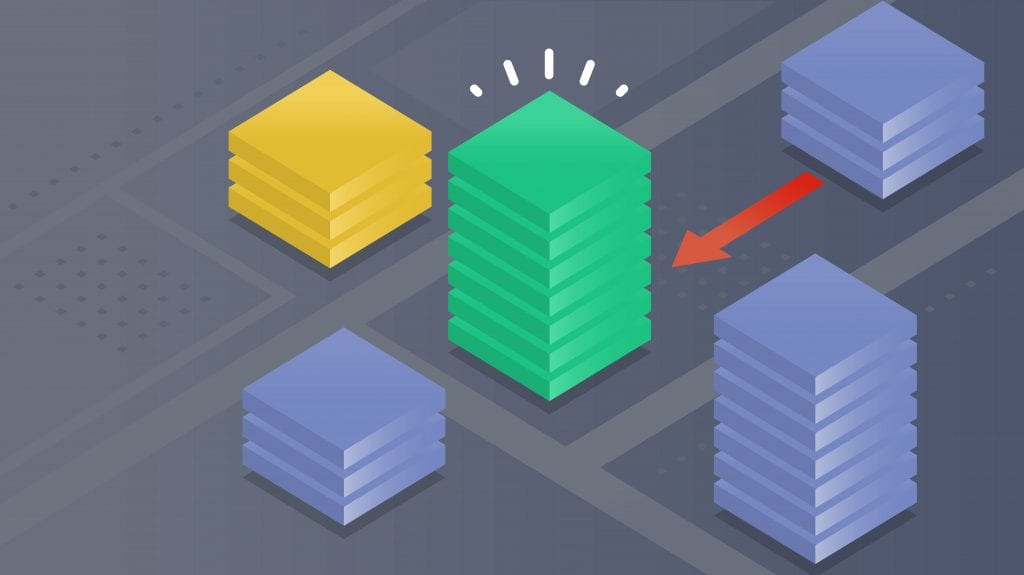
When it comes to automatic speech recognition (ASR) technology, accuracy is everything. If your ASR system’s output is riddled with errors, there’s a good chance that potential customers will seek out a different vendor.
But evaluating an ASR engine’s accuracy can be tricky, especially given that the world-changing events of the past year have shifted users’ expectations. Now users want ASR systems to understand a wide range of voices across numerous acoustic environments, from podcasts and quarterly earnings calls to live-streamed video conferences and virtual events.
Given these customer needs, it’s become clear that current ASR accuracy benchmarking methods are ineffective. This is for a few reasons:
- The most commonly used datasets contain audio files that are more than five years old, and none of them feature the wide variety of voices or acoustic environments that modern users need.
- These datasets also fail to represent today’s use cases — long-form, entity-specific audio containing both dates and numbers.
- Very few of these traditional evaluation datasets are free to use, limiting access to only larger research groups or well-funded private companies.
That’s why Rev is thrilled to announce our new, free set of tools designed to make ASR accuracy testing easier and more accessible for everyone. These tools include:
- The Earnings-21 Dataset, a brand new evaluation dataset with 39 hours of unedited, long-form audio from 2020; and
- FSTAlign, a free, open-source tool that uses text alignment to quickly calculate the word error rate in an ASR transcript.
With this release, users can evaluate the WER of any ASR vendor (yes, including Rev!) and compare them to see which best suits your needs.
A Newer, Better Evaluation Dataset
The Earnings-21 dataset comprises 39 hours of raw, unedited earnings calls from nine different financial sectors, as well as the richly annotated transcripts (with punctuation, true-casing and named entities) of each call.
Unlike traditional evaluation datasets — including sets from LibriSpeech, CallHome, and others — our new Earnings-21 dataset only contains audio from 2020. This recency is important because so many industries transitioned to remote operations during the pandemic, including jargon-heavy sectors like the financial and legal industries.
The world’s move to remote fundamentally changed the lexicons of ASR engines — audio became longer and contained much more specific terminology. Earnings-21 contains long-form, entity-dense speech in an effort to empower customers, developers, and researchers to benchmark ASR systems in the wild, paying particular attention to named entity recognition (NER).
This is also an exciting step forward for trust and accessibility in ASR. Earnings-21 provides a public benchmark four commercial ASR models, two internal models built with open-source tools, and an open-source LibriSpeech model to help mitigate speech-to-text providers calculating their WER results using the kinds of audio that they know their engine processes accurately (also known as “cherry-picking”).
An Easy, Accessible Way to Calculate Word Error Rate
In addition to our newer, more relevant dataset, we’re also providing a resource we’ve dubbed FSTAlign. This public, free-to-use tool can quickly compute WER by leveraging NER annotations. This public, free-to-use tool analyzes two comparable transcripts to decipher their differences and calculate WER.
All you have to do is input a ground-truth transcript and its corresponding ASR-generated transcript. With those inputs, FSTAlign will analyze the two, decipher their differences, and produce a WER analysis.
Unlike other text-alignment tools on the market, FSTAlign can handle long-form audio and won’t fritz out when analyzing a large file. Furthermore, FSTAlign is better equipped to calculate advanced WER on date- or number-related errors (like an engine incorrectly outputting “401k” as “four oh one kay”).
With these releases, the speech recognition community in industry and academia can push research forward with more complex audio files, and developers can see for themselves who has the best WER on the market. And, as the industry’s most accurate ASR engine, we’re pretty confident that Rev will come out on top.
But there’s only one way to find out!
Access Our Free Tools and See For Yourself!
Heading
Heading 1
Heading 2
Heading 3
Heading 4
Heading 5
Heading 6
Lorem ipsum dolor sit amet, consectetur adipiscing elit, sed do eiusmod tempor incididunt ut labore et dolore magna aliqua. Ut enim ad minim veniam, quis nostrud exercitation ullamco laboris nisi ut aliquip ex ea commodo consequat. Duis aute irure dolor in reprehenderit in voluptate velit esse cillum dolore eu fugiat nulla pariatur.
Block quote
Ordered list
- Item 1
- Item 2
- Item 3
Unordered list
- Item A
- Item B
- Item C
Bold text
Emphasis
Superscript
Subscript
Subscribe to The Rev Blog
Sign up to get Rev content delivered straight to your inbox.